Neosapience -- which is my word for artificial intelligence (AI) -- is obviously all over the news. From self driving cars to ChatGPT ( technically, large language models) this new 'technology' has not only taken the world by storm but threatens certain core financial and social constructs that define human society. There is however a counter-view that claims that neo sapiens (AI programs or silicon intelligence) can never supersede the original homo sapiens (carbon based humans and animals) because they are being 'programmed' or built by humans. Continuing on this theme, it is argued because neo sapiens are being built by homo sapiens, they are at best an imitation of the original creators and so cannot be anything new, different or superior to what the originals are. Hence, humanity is safe from a takeover by neosapience. In this article, we argue why this is not true.
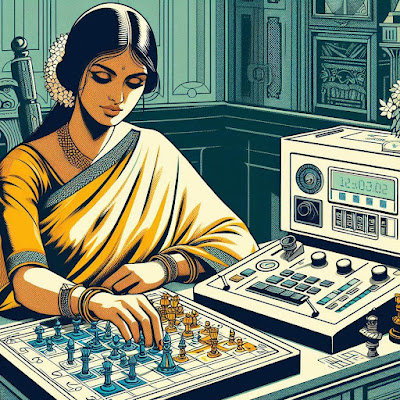
But to begin with what is intelligence? A simple, straightforward definition is unlikely to satisfy everyone so first let us define a model and then explore various models of intelligence.
What is a Model?
A model is a representation of something that 'exists' out there in the 'real' world. A model car, made of wood and plastic, mimics the behaviour of real car to a certain extent, but it can be made more realistic if we spend more money and time to include engines, tires etc. A mathematical model, like equations of motion or gravitation, developed by Isaac Newton, helps us mimic the behaviour of physical objects -- from balls to spaceships. A computer system -- like SAP -- helps us model an enterprise like Tata Steel or Hindustan Lever and tells us the money in their accounts or the inventory position in their warehouse. Building models, whether physical or digital helps us understand and mimic the world around us.
When we try to understand, mimic or model intelligent behaviour we have the choice of two broad categories of models.
Algorithmic models - that define intelligent behaviour as a set of tasks or steps that are required to, say, calculate the product of two numbers or the interest accrued in a bank account based on deposits and withdrawals, or define the steps required to solve a sudoku or Rubik's cube or even calculate the exact thrust or direction of a rocket engine that is travelling through space.
In each case, the complexity of each task is different but the model consists of breaking down the problem into smaller, easier problem and then assembling the answers in a clever manner to achieve the goals.
Non-Algorithmic models - where it is impossible to identify either a set of tasks or a 'clever' sequence of tasks that can achieve the goal. Typical examples of non-algorithmic intelligence include, for example, writing original computer programs ( to solve new problems), generating original poetry or prose or artwork that appeals to other humans and even coming up with original scientific equations ( say those that help us calculate gravitational forces). Mundane task like crossing a busy street are also examples of extreme non-algorithmic intelligence but we do not think much about them because even dogs and cats can do so!
To understand the difference between these two kinds of models let us look at two simple examples.
The gravitational model 'discovered' by Isaac Newton tells us how to calculate the gravitational forces between to massive objects ( of mass m1, m2) separated by a distance r. Since the gravitational constant G is known to, and is the same for, everyone -- even non-humans on a distant planet, anyone can arrive at the right answer.
Similarly a regression model that, say, connects the money spent on advertising to the actual sales of a product, is known, as a concept, to almost any marketing person who has learnt statistics in an MBA program. However the exact value of the two constants in the model (the slope, m and the intercept, c) changes from case to case. In the case of lipsticks, Hindustan Lever that has data on ad-spends and sales of lipsticks for the last five years, can determine the value of m and c and use that to predict lipstick sales. Similarly, in the case of cheese, Amul has the data on ad-spends and sales for the last five years and they can determine the value of m and c and predict cheese sales. So even though both Hindustan Lever and Amul knows how to use regression, HLL cannot build a model for cheese and Amul cannot build a model for lipsticks. ( And a B-school teacher like me, cannot build for anything, since I do not have any data, even though I know how to build it if I had the data)
In the case of gravitation, the model is completely defined by the equation F = G*m1*m2/r2 where {G = 6.674×10-11m3kg-1s-2 } is known to everyone. In the case of regression, the model is defined not ONLY by the equation Sales = m*AdSpend + c but ALSO by the exact values for say, lipstick : { m = 2, c =3} that is available with HLL and for cheese : {m = 20, c=3.5} that is available with Amul. The power of the model lies not in the algorithmic application of an equation but in the values of the constants, that are determined on the basis of historical data.
This set or collection of values, from the two simple pieces in linear regression {m,c} to the trillions of pieces in ChatGPT, is what defines these models.
Models of Intelligence
Initial attempts to model human intelligence, as in playing chess or translating from English to Bengali, were based on algorithmic models and had very limited success. However quite a few smart people caught on to the fact that the human brain is not algorithmic and intelligence lies, not in any of the neurons in the brain but in the way each simple neuron in the brain is connected to, or influences, the other neurons. But since there are nearly 100 billion neurons in each human brain, determining the influence of each on all the others was an insurmountable computational problem. The two key algorithms -- the backpropagation algorithm and the stochastic gradient descent algorithm - that help us to calculate the influence (collectively referred to as weights w, and biases, b ) have been known since the 1980s, but no one had the data or the computational power to build a non-trivial model by determining the exact values of the numerous {w,b} parameters.
The situation changed dramatically with the arrival of BigTech companies ( Google, Amazon, Meta etc.) with their voracious appetite for consumer data and new hardware (for example, GPUs from NVidia and cloud based systems from Amazon AWS). Now, for the first time, it was possible to analyse trillions of data points and calculate the billions of values that define the new "models".
As an aside, widely used machine learning techniques like regression, classification, clustering are not based on the architecture of the human brain but on principles of statistics. However the models that are created using these techniques consist of a collection of parameters whose values are determined from the set of historical on which these statistical techniques are applied. Once again, the strength, or quality, of the model lies not in the algorithm or the technique but the data on which the algorithm or technique is applied. However, all such statistics based models have been surpassed by a new class of algorithms that mimic the behaviour of the human brain.
The technology architecture of artificial neural networks ( ANNs) can now simulate, with software, the structure of the human brain with increasing levels of sophistication. The "architecture" in this case refers to how the simulated neurons are deemed to be connected to, and influence, each other because this, in some mysterious and ill understood way, reflects on the nature of problems that can be solved.
The initial feed-forward architecture was good for a large variety of problems but there are others, like convolutional networks and reinforcement networks that were found to be better for image recognition and text analysis. The current superstar in this area is one that is referred to as 'transformers' (nothing to do with alternating currents) that are based on 'attention' and the next one on the horizon is based on 'graphs'.
The techniques used to build, or simulate, these networks and the algorithms needed to calculate the parameters are all in the public domain. So in principle anyone can build these models if -- and only if -- they have the behavioural data from thousands of millions of individuals and the computation power to process them and calculate the values of the trillions of parameters that are needed by the model. At present, only big conglomerates have the ability to do so. The rest of us can only watch from the sidelines and only hope to use these models if we can afford to access them, as it happens in the case of ChatGPT.
Surpassing Humans
Now that we have some idea of what these models are, let us circle back to the question of whether these models can demonstrate behaviour that is better, superior, or more intelligent, than that of its creators. One of the biggest mysteries surrounding these models, is that even though the algorithm used to generate these trillions of numbers is known, the exact reason why a particular parameter has a specific value is indeterminable. There is no way to connect a cause -- say the image of fat man in a crowd -- to any effect, that is the value of a specific parameter. Since everything is probabilistic, it is impossible to identify a chain of causality. This leads to two kinds of behaviour. First, we have systems that hallucinate or generate illogical responses and second, we have systems that generate output that are logical and correct but have never been seen in humans before. This second behaviour has been detected in chess playing systems that have come up with novel strategies that are completely unknown to even the best of human chess players. [As an aside, no human, not even the best of the lot can win against any chess playing program today]
The key takeaway from this situation is that the strength or quality of any model does not lie in the algorithm or programming skill of the person who built it but on the quality and quantity of the data that is used, or ingested, while training the model. That is why it is incorrect to assume that neo sapient systems can never supersede the ability of homo sapiens, who build them.
The process of learning and its outcome does not depend on the competence of the teacher, but on the way the student can apply it to the environment in which they find themselves. Had it not been the case, Einstein and Newton would not have been able to generate knowledge or insights that were not available with their teachers.
Today, large language models like ChatGPT and others, can write computer programs, poems, stories, screenplays and generate images and videos and the quality is improving by leaps and bounds with every passing day. In the case of business communication and computer programs, areas where LLMs have had access to maximum data, they are already better than 99% of humans. [ For example, the graphic used in this post was created by me with Bing in about 15 mins and I am sure that a vast majority of my readers would not be able to create anything similar on their own, without using a generative AI tool ] Salman Rushdie has claimed that in the case of originality of thought and humour AI or neo-sapient artifacts are still deficient but this claim is essentially baseless because with the passage of time and the availability of more and better data the capability can only increase.
Physics puts an upper limit on the speed that a material body can travel at and this is the speed of light. To go faster than this limit, one has to conjure up strange artifacts like tachyons that lie beyond the realm of normal physics. Similarly, is there some divine or extra-human power that allows some of us to demonstrate creativity that no one else can replicate? If -- and only if -- there is, then our current crop of neo sapients would never have the ability to access that kind of power and and hence would never equal or surpass these highly gifted humans. But if there is nothing divine in human ability, then there is nothing that can stop neo sapients from surpassing homo sapiens in any realms of intelligent behaviour.
Post Script : Genetic Information Models
If we consider genetics, then there is another -- possibly controversial and certainly non-mainstream -- analogy that can be brought to bear in this debate. While the debate between nature and nurture -- whether we are born with certain abilities or whether we acquire them in our life -- is still open and contested, we do know for sure that humans are more intelligent than, say dogs or cats, and this because of our genome. The genome of a living organism is actually a sequence of proteins grouped into genes and arranged on our chromosomes. This is basically information. So our intelligence is based on information stored in our genes and this can be viewed as the model. The process -- or algorithm -- that converts this information into proteins that make up our body is almost the same for all living things, so the magic lies in the information stored in the model and not in the process of converting it into our material body. But unlike human and current machine learning models where this information pattern is created rapidly, the genetic information gets created or updated very slowly over many generations and millions of years. Nevertheless, it is still information ( or data) that plays the key role in the ascent of species. Except that biological sapients have been evolving far more slowly than our machine counterparts. But that is a different story altogether.